Demystifying the Digital Twin for Power & Utilities. Time to Rethink the Definition?

“We have a lot of data, but we still don't have a lot of information”
If this sounds familiar, you’re far from alone. Utilities today continue to struggle with the journey to efficiently operationalize data from around the organization into repeatable business value. It’s not for lack of trying; digital strategy is in place and teams are being structured to meet these challenges, yet the conversion point from data into valuable information still seems to be more manual than automatic.
Put simply, data really can’t turn into information without context. In operations, data must be anchored to its appropriate place in the process – usually to an asset hierarchy, process diagram, or even a network. Skilled subject matter experts understand this context natively, having developed it over time. But for many employees, analysts, and data scientists, this data exists in various formats and silos across the organization, most often lacking the quality, the context, and the relationships that make these individual points valuable to operations. This translates into longer time for analytics and slower digital deployment cycles.
Read also: What is a digital twin and how does it create value in industry?
The development of new digital assets is often initiated by utility innovation programs, but the path of scaling and embedding digital assets in operations is vague. For example, there is already a well-defined operating model for planning, construction, and maintenance of utility assets such as transformers and wires. However, the operating model for digital assets in many utility organizations is yet to be defined. A clear definition for digital assets is critical to realizing their full potential and value.
Enter the digital twin. Since their inception as a concept, digital twins have been an excellent method for presenting data in context and bridging the gap between the physical and digital world. Most often used for asset performance management (APM) use cases, digital twins are in control rooms today for simulating what-if scenarios and for enabling remote operations.

Figure 1: The generally accepted concept of a digital twin with a subset of data in context
On the surface, a digital twin of an asset seems rather straightforward from a user perspective: relevant time series data for an asset is tied to the various subcomponents and areas of interest. That data is then presented with a visual model of the asset along with health indicators based on analytical models and simulators. This can give a more comprehensive view to the asset’s current state and help engineering teams make better maintenance decisions.
Evolving the definition of a 'Digital Twin'
For a greener, more reliable, more secure energy future, the utility industry must make digital twins a ubiquitous part of everyday operations. But what does this mean, and how does it look? It starts with re-defining the digital twin; away from rigid, constrained, legacy concepts and towards the idea that they must be living, adaptable, scalable, and manageable. Digital twins can be used to represent entire systems of interconnected systems in addition to assets. In this case, think of entire industrial sites with physical properties and assets, networks of infrastructure and power flows, and even a digital twin of the enterprise. Thinking about a digital twin in this way opens new use cases around autonomous site operations, advanced predictive maintenance, streamlined planning, site-to-site performance benchmarking, and improved connection analysis, among many others.

To solve this expanded view of use cases, it may be more appropriate to think of a digital twin as the complete underlying canon of data and information that can be accessed and understood by any data or engineering user. The traditional scope of data (time series and simulators) must grow quickly to include other non-traditional data sets such as work orders, images, CAD models, etc. This thinking takes advantage of the 90% of data that still goes unused by power and utility organizations and puts it in context with new and increasingly valuable layers of granularity.

Read also: The digital twin: the evolution of a key concept of industry 4.0
While the future opportunity to adopt and leverage digital twins for more business processes is significant, there are some challenges that must be addressed in both the technology layer and in the operating model.

Embedding digital twins in utility business operations can be opaque:
A digital twin is not inherently valuable if it isn’t being used to change and evolve a business process or workflow. Today, digital twins (in their limited definition) are commissioned with unclear business cases and metrics, making it difficult to calculate true ROI of the project. Future owners and end users may also not be fully engaged in the project or able to trust the data. As a result, releasing the digital twin from digital innovation programs and embedding operations could face the risk of limited adoption or integration in utility asset management or maintenance processes.

Industrial data is inherently messy and untrusted:
Rarely does an organization have a data model and infrastructure that is readily fit for this new definition of the digital twin. Digital twins today have context that is all derived manually through subject matter expertise. In order to scale digital twins, this context mapping process needs to change to a more automatic, yet transparent, approach that enables more trust in the data to enable new ways of working in utility operations.

Costs to deploy and scale are still perceived to be prohibitively expensive:
Even with significant technology advances, a digital twin project is still perceived to take significant resources and months to accomplish. This is one reason why so many projects get stuck at the proof of concept; the operational value may be clear but there’s no visible path to reducing the costs for each subsequent digital twin model deployment in utility operations.
Understanding the relationship between Industrial Data Operations and a well-executed digital twin strategy
To address the challenges of perceived high cost, untrusted data and business operations integration, a digital twin strategy requires appropriate industrialization of the underlying data management, business process innovation, and change management to drive both efficiency and scale.
Learn more: Contextualized data and digital twins amplify digitization value
The underlying costs of data management and contextualization must come down through automation. This market need has delivered innovation in the field of industrial data operations software, which aims to streamline the process by which complex, raw data gets transformed into business value. Put another way, industrial data operations enable more effective provisioning, deployment, and management of digital twins at various levels of granularity.
Operational Digital Twin
The operational digital twin provides the foundation needed to build tailored solutions across domains. Cognite helps utilities scale solutions, reduce time-to-value, and establish trust in solutions by automating the process of creating relationships across previously siloed data. Cognite's unique AI-powered contextualization services provide the foundation to make data self-service for use in tools and applications your teams are already know.

This step-change in efficiency is a result of tooling and automation in the following areas:
- Data integration: where data from any source system can be accessed and provided with certain quality assurance for data modeling
- Data contextualization: where relationships between different data sets are formed in code and kept track of over time in an industrial knowledge graph.
- Application development: through tools and microservices that make data useful to a range of data consumers including data scientists, analysts, and engineers.
- Use case management and scaling: where common data products and templates can be leveraged repeatably to scale across similar assets, sites, and analytical problems.

Where to start?
It may be overwhelming to think of the digital twin development process in its entirety, but breaking it down into concrete steps and milestones makes it much more approachable. The goal is, after all, to deliver incremental value from digital transformation that snowballs into significant ROI and a step change in how work is activated and performed. Just like Google Maps puts consumer data into context and continues to get better with more features and more context, an industrial digital twin approach must likewise be additive and act as a platform for continuous improvement for utility operations.

Zero in on real business problems:
What is the right use case that has a well-defined problem statement that can be traced back to real tangible value? Where is there immediate need for new operational visibility? Where do you expect to need more of it in 3-5 years? Here is where it pays to be thinking about digital twins like a product manager with both short and long-term vision.

Iterate pragmatically in the data layer:
Industrial data will never be perfect and it doesn’t have to be. Start building your digital twin with data that is used in many use cases and solve the business problem at hand before integrating new sources and augmenting the use case. Digital is as much about continuous improvement as it is about the tech stack, with new tooling that makes it easier to grow your digital twin footprint over time.

Empower your experts:
When data becomes accessible to the wider enterprise via the contextualized digital twin, all stakeholders benefit. It no longer takes 2-3 months of back and forth on a project to develop a new algorithm or insight based on the data. Instead, dashboards and analytics can be created and then scaled in an afternoon. True digital change and innovation is a product of data democratization and improved trust.

Think end to end - digital twin excellence:
Digital twins empower utility management, engineers, and data scientists to increase asset performance and drive process excellence. Understanding how a digital twin will impact the way you plan, construct, operate and maintain assets - or a system of assets - in the future will be critical to drive a digital twin that enables operational excellence.
Looking to jumpstart, accelerate, or pivot your strategy and approach to digital twins? Together, Tetra Tech and Cognite enable utilities to increase resilience and drive decarbonization with digital twin-based technology and practices for predictive maintenance, digital inspections, and system analytics. Let’s start the conversation.
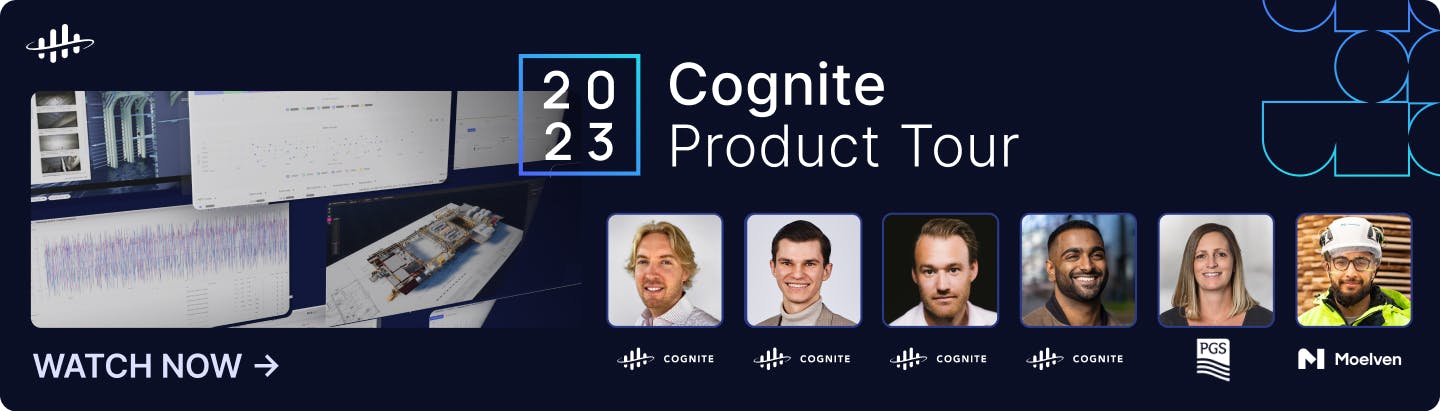
Gabe Prado, Cognite, Sr. Director of Product Marketing, Power & Utilities
Georg Baecker, Tetra Tech, Sr. Director, Utility Management Consulting Leader – North America
About Cognite:
Cognite is a global industrial SaaS company that was established with one clear vision: to rapidly empower industrial companies with contextualized, trustworthy, and accessible data to help drive the full-scale digital transformation of asset-heavy industries around the world. Our core Industrial DataOps platform, Cognite Data Fusion™, enables industrial data and domain users to collaborate quickly and safely to develop, operationalize, and scale industrial AI solutions and applications to deliver both profitability and sustainability. Visit us at cognite.com and follow us on Twitter and LinkedIn.
About Tetra Tech:
Tetra Tech is a leading provider of high-end consulting and engineering services for projects worldwide. With 21,000 associates working together, Tetra Tech provides clear solutions to complex problems in water, environment, sustainable infrastructure, and renewable energy. We are Leading with Science® supported by our Tetra Tech Delta technologies and analytical tools to provide sustainable and resilient solutions for our clients. For more information about Tetra Tech, please visit tetratech.com or follow us on LinkedIn, Twitter, and Facebook.
See Cognite Data Fusion® in action
Get in touch with our product experts to learn more and identify quick wins