Open Industrial Digital Twin

The industrial digital twin: A practical definition focused on driving industrial innovation and value
The idea of digital twins came from the manufacturing world nearly 20 years ago; more specifically, from a 2002 article in an academic journal that talked about 'the idea that information about a physical object can be separated from the object itself and then mirror or twin that object.'

We know the digital twin as a combination of one or more data sets and a visual representation of the physical object it mirrors. But while the digital twin concept is no longer new, its meaning continues to expand based on technological advancement, particularly in the realm of the Industrial Internet of Things (loT).

Today, digital twins are morphing to meet the practical needs of users. In asset-heavy industries, optimizing production, improving product quality, and predictive maintenance have all amplified the need for a digital representation of both the past and present condition of a process or asset.
Gartner predicts that 'by 2023, 33% of owner-operators of homogeneous composite assets will create their own digital twins, up from less than 5% in 2018' while 'at least 50% of OEMs' mass-produced industrial and commercial assets will directly integrate supplier product sensor data into their own composite digital twins, up from less than 10% today.'
Garter further indicates that digitalization will motivate industrial companies to integrate and even embed their digital twins with one another to increase their own competitiveness. To do that, industrial companies need to make sound strategic decisions now to lay a trusted and flexible data foundation for success.
Building the industrial digital twin
To enhance the overall understanding of their operations, industrial companies will need an industrial digital twin that contextualizes data across their entire OT, IT and engineering data spectrum. Here's our definition:
What is an industrial digital twin?
An industrial digital twin is the aggregation of all possible data types and data sets, both historical and real-time, directly or indirectly related to a given physical asset or set of assets in an easily accessible, unified location.
The collected data must be trusted and contextualized, linked in a way that mirrors the real world, and made consumable for a variety of use cases.
Digital twins must serve data in a way that aligns to how operational decisions are made. As a result, companies may need multiple twins, as the type and nature of decisions are different. A digital twin for supply chain, one for different operating conditions, one that reflects maintenance, one that's for visualization, one for simulation—and so on.

What this shows is that a digital twin isn't a monolith, but an ecosystem. To support that ecosystem, industrial companies need an efficient way of populating all the different digital twins with data in a scalable way.
This next frontier in the digital twin decouples individual models (for example, applications, simulation models, and analytics) from separate source systems, eliminating the unnecessary complexity established in point-to-point integrations.
Evolving the digital twin
Equipping the process & capabilities that bring digital twins to life

Building your digital twin requires turning siloed data sources into trusted, contextualized data for all. This includes integrating structured and unstructured data, making sure there is sufficient trust and quality in the data, accelerating data modeling, and providing data governance—all while templatizing repeatable tasks across the activities above.
In addition to the data layer, it's just as important to have technical capabilities that bring digital twins into live operations. This includes functionality around 3D visualization, building analytic models, open APIs, using unstructured data, and even capabilities for robotics.
With the foundational data elements in place, bringing a digital twin to life requires leveraging technologies around visualization, data science, dashboarding, and more. This is the functional set of tooling that creates an intuitive representation from the underlying data foundation. Cognite's offer is unique because our technology incorporates both the data layer and these functional toolkits to provide a robust launching pad for open industrial digital twins.
Suite of specialized digital twin tools for ongoing enrichment
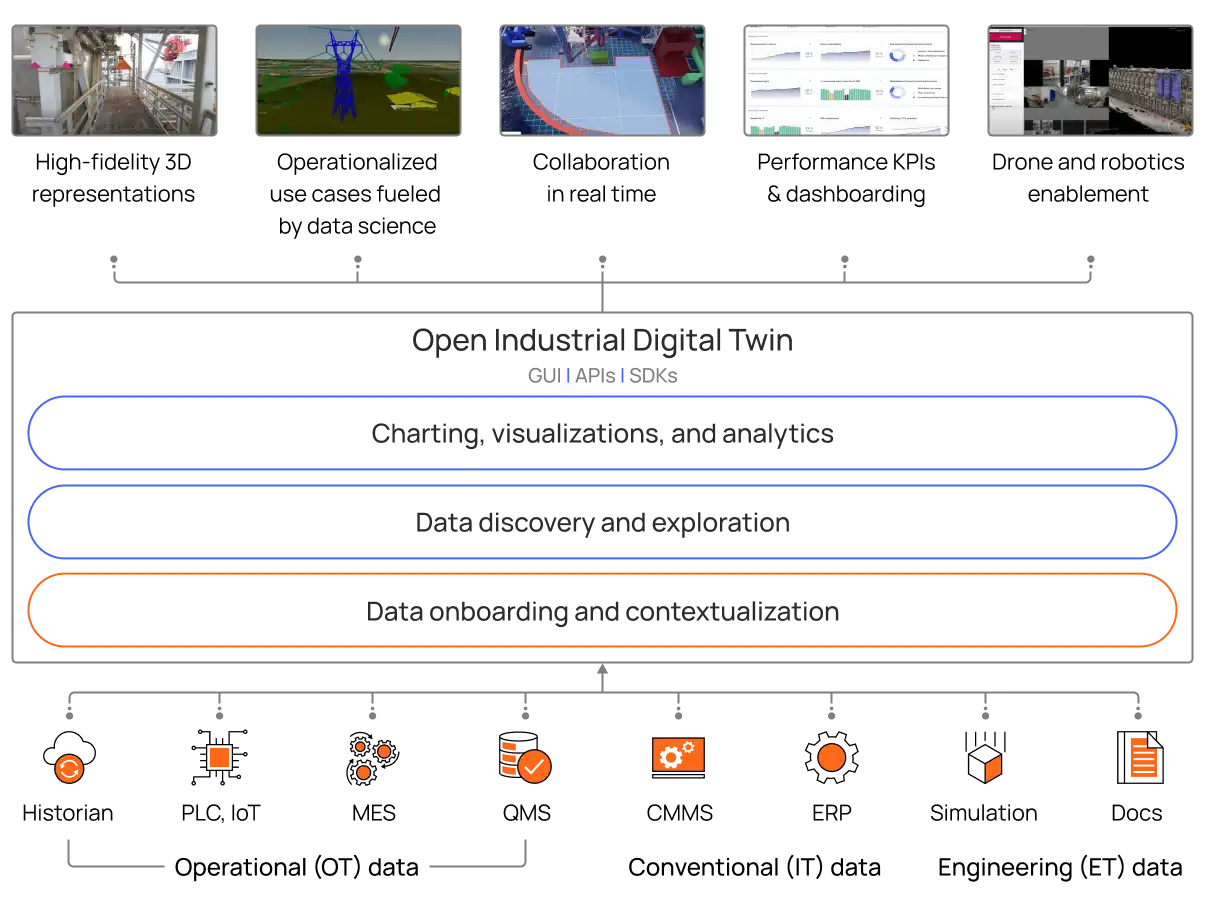
Digital twins can give a more comprehensive view of the current state of assets or processes and also be extended to represent entire systems of interconnected systems in addition to assets. Thinking about a digital twin in this wav enables new use cases around energy optimization, advanced predictive maintenance, streamlined planning, site-to-site performance benchmarking, and autonomous site operations among many others.
To solve this expanded scope of use cases, a digital twin must turn data into information that can be accessed and understood by both data and operational experts. The traditional scope of data (time series and simulators) must expand to include other nontraditional data sets such as work orders, images, and CAD models for more complex use cases like real-time process monitoring with computer vision.
Examples of data types that Cognite Data Fusions liberates and fuses
Time seriesE.g., readings of temperature, pressure, speed, torque, direction and number of occurrences.DocumentationE.g., component descriptions, service manual and engineering diagrams.SequencesE.g., operating curves, tabular data, multivariate data.EventsE.g., system failures, identified abnormalities, planned maintenance and conducted inspections.Visual dataE.g., 3D model of entire vessels or components, images of individual parts or image to document defects.

While many companies have undertaken digital twin initiatives, few have been able to provide value at scale. One of the biggest pitfalls is a monolithic approach to digital twins that struggle to scale beyond an initial proof of concept. To achieve scalability, an open, interoperable approach is needed.
An interoperable approach can be simplified to a data layer, an analytics layer, and a low-code layer for visual app development. More formally, this translates into an Industrial DataOps layer, a ModelOps layer—including simulation hybrids—and a DevOps layer.
Industrial data operations makes industry 4.0 work.

For these layers to work together in a composable fashion, solutions will require openness and interoperability. For example, as business needs change, new best-of-breed applications and tools may be required for analysis.
Additionally, different low-code application frameworks—and SaaS applications outright—may be chosen for different business functions and their users. These applications must be independent of the underlying data layer.
Industrial customer requirements for high-impact asset optimization solutions are highly heterogeneous or require significant customization.

The open industrial digital twin makes the contextualized data available to the user. To operationalize use cases—seen as the DevOps layer in the image above, focusing on the application development itself—we need full confidence in the data presented to the application users.
We’re talking about trust in data. This is a necessity, otherwise users can not use the digital twin to improve workflows and capture business value.
Achieving autonomous operations is a journey with value capture along the way.

Trust—or confidence—in data accuracy becomes more critical as we advance into predictive and prescriptive solutions, and ultimately all the way to closed-loop decision execution. While the latter is years into the future, trust in data is fundamental to the journey toward autonomy.
If we want to execute at scale, we need industrial data management that provides a robust, well-governed, high-confidence “IT-like” environment. We need high-quality, verifiable, observable data and model management. In other words, our digital twin platform needs to have a strong DataOps backbone.

Trustworthy data delivery in a real-time environment to many different data consumers—this is what the Industrial DataOps concept is all about. To operationalize digital twins in the industry, we need trusted data delivery, focused on continuous data integration across all OT, IT, ET, and visual data sources.
With an efficient way to create digital twins, companies can enable more people across the enterprise to make data-driven decisions, putting the power of digital innovation into the hands of experts at plant floor operations, remote, across plants, in aftermarket services, and in R&D.
Without a robust foundation of Industrial DataOps, trust in data, data models, and data-driven recommendations remains low, resulting in the inability to progress into scalable operations improvement.
Industrial DataOps for digital twins
A digital twin isn’t a monolith, but an ecosystem. This composable way of thinking about the ecosystem of digital twins supports how businesses in general need to be run across engineering, operations, and product.
What is needed is not a single digital twin that perfectly encapsulates all aspects of the physical reality it mirrors, but rather an evolving set of “digital siblings.” Each sibling shares a lot of the same DNA (data, tools, and practices) but is built for a specific purpose, can evolve on its own, and provides value in isolation.
The family of siblings accurately reflects the various aspects of the physical reality that provide value, and provide more autonomy and local governance than the building and maintenance of a single canonical digital twin.
This puts the spotlight on what’s blocking enterprise business value today: How can we efficiently populate and manage the data needed for the digital twins across the inherent entropy and ever-changing nature of physical equipment and production lines?
The answer lies in how to think about the data backbone supporting the digital twins. The data backbone needs to be run in a distributed but governed manner known as Industrial DataOps.
To adequately extract the value of industrial data insights, operationalizing data must become a core part of your business strategy. This translates into developing and scaling mission-critical use cases. Data must be made available, useful, and valuable in the industrial context.

Industrial DataOps enables more effective provisioning, deployment, and management of digital twins at various levels of granularity. This step change in efficiency is a result of tooling and automation in the following areas:
Make data available: Data from any source system can be accessed and provided with certain quality assurance for data modeling.
Make data meaningful: Relationships between different data sets are formed in code and maintained in an industrial knowledge graph.
Make data useful: Tools and microservices that make data useful to a range of data consumers, including data scientists, analysts, and engineers.
Make data valuable: Where common data products and templates can be leveraged repeatedly to scale across similar assets, sites, and analytical problems.
Unpacking the operational digital twin
The makings of the open industrial digital twin
“Cognite Data Fusion® creates contextualized data models that abstract away source system complexity, enabling customers to quickly and reliably build new analytics dashboards, new production solutions, and digital twins and to scale these across industrial assets”.
Forrester
To efficiently accelerate the initial rollout and the incremental improvements for digital twins, team engagement and enablement is a requirement. It’s necessary to engage and build on the competency of the operators and subject-matter experts who know their equipment and production lines the best, and to drive efficient data-grounded collaboration for these roles.
.webp&w=1920&q=75)
The data backbone to power digital twins needs to be governed in efficient ways to avoid the master data management challenges of the past—including tracking data lineage, managing access rights, and monitoring data quality, to mention a few examples. The governance structure must focus on creating data products that can be used, reused, and collaborated on in efficient and cross-disciplinary ways. The data products must be easily composable and be constructed similar to how humans think about data - where physical equipment are interconnected both physically and logically.
Cognite Data Fusion®, the leading Industrial DataOps platform, unlocks the potential of industrial digital twins powered by data transformation and automated contextualization services for developing and maintaining a comprehensive, dynamic industrial knowledge graph. The industrial knowledge graph acts as the foundation for the twin and provides the point of access for data discovery and application development.
Using Cognite Data Fusion®, industrial organizations are improving brownfield asset performance using digital twins of equipment, assets, and processes.

By integrating live operational data from work orders, engineering drawings, images, asset hierarchies and more sensor networks historical data, the industrial digital twin delivers the complete industrial data spectrum in one accessible user interface and API, enabling:
- Data that can be shared and acted on
- Advanced, easy-to-access insights for all data consumers
- Predictive maintenance
- Production optimization
- Improved safety
- Faster responses to adverse events
Develop, operationalize and scale use cases 10 times faster
For the high-value purposes of data science model development, the value of interconnected industrial data made possible by the data integration layer of the digital twin doesn’t depend on any 3D visual data representation. It’s all about having an interconnected data model that can be queried programmatically.
What industrial data contribute to our understanding of our operation

Software developers—and data scientists by extension alike—all depend on data models to make analytical software applications work and scale.
For example, take someone developing a production optimization application across a fleet of assets. What they need is a strong domain API that gives them instant access to the data model, which contains all the relevant data for any given asset or process—regardless of where that data comes from or where it’s stored. Also, they need it in domain language, not in the language of databases.
To provide this level of convenience to the developer and data scientist, the output of the work taking place at the data management and data integration layer of a digital twin architecture is a data model (or a set of data models) that removes the data complexity. This enables developers and data scientists to focus on application logic and algorithm development itself—not on finding, transforming, integrating, and cleaning data before they can use it. In short, the backbone of all digital twins is a unified, developer-friendly data model.
Cognite Data Fusion delivers an industrial knowledge graph to develop, operationalize, and scale use cases

A scalable approach to modeling data
Cognite Data Fusion® an Industrial DataOps platform, supports hybrid data integration for both streaming and batch-oriented sources. Connect to all OT, IT, and ET data sources with a high-performance historian and OPC-UA/MQTT connectors, and use Spark-powered on-platform transformation services for data pipeline streamlining.

Data contextualization involves connecting all the data for a clearer understanding of an asset or facility. Cognite Data Fusion® delivers contextualized data as a service through a combination of AI-powered contextualization services and subject-matter expert enablement. Convert data to knowledge by setting up contextualization pipelines to populate the Cognite Data Fusion® industrial knowledge graph. Make data available, meaningful and valuable independent of its origins. Empower all data consumers with instant access to past, real-time and synthetic (simulated and predicted) data and scenarios. Simple access to complex industrial data.
Fully contextualized operational data can only be provided by an industrial knowledge graph with automated population.

Create a model for one asset, then scale it across an entire installation. Spend less time, effort, and resources on finding, cleaning, and mapping data, and more time on generating value. The step of building a reference data model should be a standard part of moving data from sources to applications within your data architecture, as it will represent the beginning of a reusable data asset.
Once a reference data model has been defined, we can move to execution and scaling. Rapid development and scaling are made possible by autocompleting solution-specific use case data models by applying AI-powered pattern recognition and reinforcement learning. Use case templatization encapsulates much of the application complexity directly at the data layer, making data visualization scaling from one to thousands of dashboards or analytic models possible in a matter of hours.

Maintaining an industrial digital twin
For data to be operationally used at scale, especially for critical operations, it needs to be trusted. For data to be trusted, it needs to be productized. Build trust and collaborate with data consumers through data products, that may be used, reused, and collaborated on in efficient and cross-disciplinary ways.
Simply, a data product is a packaged data set, a sub-set of the entire industrial knowledge graph, used to solve a specific set of use cases. With a data product, provide transparency toward data quality, uptime, Service Level Objectives (SLO), and compliance with decentralized (owned by the domain users) governance rules, lineage, and revision auditing.
To productize your data, focus on the most valuable operational data domains first, not on the enterprise-wide master data landscape all at once. The goal is to shift from a centralized data team, such as digital or data center of excellence, into a collaborative setup, where each data domain is co-owned by the respective business function producing the data in their primary business tools.
After all, it’s the business operations team that understands the data in context best and is therefore best placed to communicate and use the data to solve operational challenges. One way of looking at governance is by separating the layers of governance structures and associated data management:
- Digital twin solution-specific data onboarding and governance, where operators and plant IT workers create data products that power operational decisions.
- Discipline-specific data onboarding and governance (for example operations, engineering, or product), where subject-matter experts and domain specialists create shareable data products.
- Enterprise-wide data onboarding and governance, where specialized data management and IT teams create enterprise-wide data products.
.webp&w=1920&q=75)
For each of these data products there’s a custodian shepherding the process. An enterprise-wide network of custodians is important to achieve the nonlinear scaling effect across sites, production lines, and equipment.
The data product owners need to ensure their data is discoverable, trustworthy, self-describing, interoperable, secure, and governed by global access control. In other words, they need to manage their data products as a service, not as data.
It’s essential to move from a conventional centralized data architecture into a domain data architecture (also referred to as a data mesh). This solves many of the challenges associated with centralized, monolithic data lakes and data warehouses. The goal becomes domain-based data as a service, not providing rows and columns of data.
.webp&w=1920&q=75)
The open industrial digital twin allows for data consumption based on the use case. Any model the user creates can live off the streaming live data that exists in the twin, enriching the twin with users developing insights (for example synthetic temperature or flow information created by a simulator for equipment where no real sensor exists) back into the twin.
Combined with the complete data spectrum, these insights on equipment behavior enhance the industrial digital twin, creating a positive feedback loop that is even more useful for future solutions.
Delivering scalable solutions with the open industrial digital twin
Digitalizing how businesses are run isn’t about what technology can do—Industrial DataOps is about having making it easy for everyone in an organization to create data products and make data-driven decisions. Convenience is the key concept to embrace. To get this distributed model to work, an Industral DataOps solution should:
- Define and update what data should be added to data products and how it relates to business needs from digital twins and solutions.
- Define and monitor who should be able to access the data and who could be interested in it.
- Define and monitor what data quality can be expected from the data in the data product.
- Define how the data product is related to other data products.
- Keep communicating with the data custodian network to make sure that innovation and collaboration happens in efficient ways across the different data domains.

In practice, every solution has specific expectations and requirements to how data is supposed to be structured and modeled, and to this end, Cognite Data Fusion® provides a flexible data modeling framework that allows for such different perspectives into data to be clearly described.
While the source data model liberates data from a variety of source systems it also maintains query ability in Cognite Data Fusion® through the same API interfaces. The domain data model synthesizes information across data sources and allows for a detailed description of an asset or process that can be continuously enriched as new information and data sources become available.

A solution data model imposes a schema that the application can validate and rely on and the instances of which are populated with the help of Cognite’s contextualization services in an automated way, leveraging a domain-guided machine-learning approach. Compared to the domain data model, which allows for a higher level of entropy and the representation of evolving ontologies, the solution data model is much more rigid while at the same time allowing for true scalability across two dimensions:
- Scalability of one Solutionis ensured through automated population of solution data model instances, e.g. scaling a maintenance optimization solution across the entire asset portfolio.
- Scalability across a portfolio of solutionsis enabled by immediate access to a wide range of data sources and the fact that application requirements to data are decoupled from the representation in the domain data model. This allows for use cases to be solved that require different levels of data granularity, e.g. plant-level maintenance optimization vs enterprise-level strategic planning. Cognite Data Fusion® serves as the data backbone for digital twins for dozens of industrial companies around the world. The SaaS product serves data to a broad set of digital twin types in a way that secures rapid rollout to hundreds of sites, makes them efficient to deploy and operate, and secures support for lean processes when they’re in production.
The open industrial digital twin in action
Enable agile manufacturing with an open industrial digital twin
Cognite has supported Elkem Carbon with their first step towards becoming a more data-driven company
The goal of the project has been to equip Elkem Carbon with machine learning tools in operations and the necessary capabilities to utilize them; to build a first version of Elkem's Carbon data model and; to demonstrate how this built data model could bring value to Elkem Carbon by solving a specific machine learning use cases in the milling step of the ramming paste production process.
Problem statement
One key source for producing off-spec material is poor conditions of the mill. This can be caused by, e.g., wear, clogging or air leakage. In the worst case, the bad state of the mill can go unnoticed for a long time if the process engineers do not monitor the system at all times, or if the fault is hard to predict from visual inspection of the data (i.e., caused by ‘sudden’ errors). Since the anomalous behavior is not detected in due time, it can lead to off-spec production and loss of revenues. Developing a state detection model was the first step in mitigating this problem.
Value
The State Detection model improves transparency on actual conditions of milling system machine(s) and its subcomponents. Looking at comprehensive data insights and KPIs, as well as utilizing the machine learning model with actionable insights:
- Enables operators to proactively act before a potential failure occurring rather than after
- Reduces the likelihood of having off-spec products
- Provides better understanding of system behavior allowing better monitoring and more advanced analysis
- Reduces the likelihood that the Mill becomes a bottleneck
Going forward
Elkem Carbon Fiskaa is operationalizing the ML tools and use cases to a larger user base. Liberating data from the rest of the production processes and related data sources will allow Elkem to capture value not only from the current Vertical Mill but a so other critical production processes.
Key project deliveries
Cognite equipped Elkem Carbon with machine learning tools utilizing contextualized data from Cognite Data Fusion®.Cognite then empowered Elkem to use the ML tools and to build one State Detection Model targeting the mill, visualized in one dashboard. At the end, the project delivered, amongst other:
- A proven and trusted State Detection Model
- Three different user-specific Grafana Dashboards for operations, maintenance and process engineers
- Live, enriched contextualized data available in Cognite Data Fusion®
- Data Science toolbox for future model development
- End-user ownership of end-solution to continue improving and iterating the end solution over time
- Foundation for future scaling across Elkem organization
- Increased potential for future cost saving at the mill

Elkem Carbon is equipped with an extensive toolbox to continue exploring data and adopt existing state detection models based on new findings
The industrial digital twin is not a single use case
The industrial digital twin is not a use case. It is not an app. It’s a platform on which to build, deploy, and scale many different use cases and applications, from those large, complex, and visually impressive to more modest on the surface, focusing on delivering actionable information to drive process improvements.
An industrial digital twin is one tool that industrial companies can use to reach a greater level of understanding and control regarding their data and their operations, discovering insights to optimize operations, increase uptime, and revolutionize business models.
Historically, a digital twin has had a single dimension of contextualization to solve the use case it was specifically created to answer. When creating an industrial digital twin, the richness of the data describing the industrial reality is critical to create many more correlations between data sources.
The industrial digital twin must be dynamic, flexible enough to meet the needs of a growing variety of users and models. This is why contextualization is crucial to the creation of the industrial digital twin, making it flexible enough and scalable enough to handle the complexities of current operations and to anticipate the demands of data-driven operations in the digitalized industrial future.
The solutions that are built on top can support personas across the organization, including subject-matter expert chart tools, applications for operations, quality, and maintenance, and the ability for data science teams to build predictive or prescriptive solutions.
Companies that deploy an industrial digital twin will finally have true control over their data—the ability to understand where it comes from, how reliable it is, and how to enrich it over time. They will also be the first ones to scale successful solutions on top of that data, which must be the priority of any digitalization initiative.
Bring AI-software capabilities into the process without replacing current applications and source systems. Build, operationalize, and scale hundreds of use cases.
See Cognite Data Fusion® in action
Get in touch with our product experts to learn more and identify quick wins