The Imperative Role of Knowledge Graphs in Generative AI for the New Era of Industrial Operations
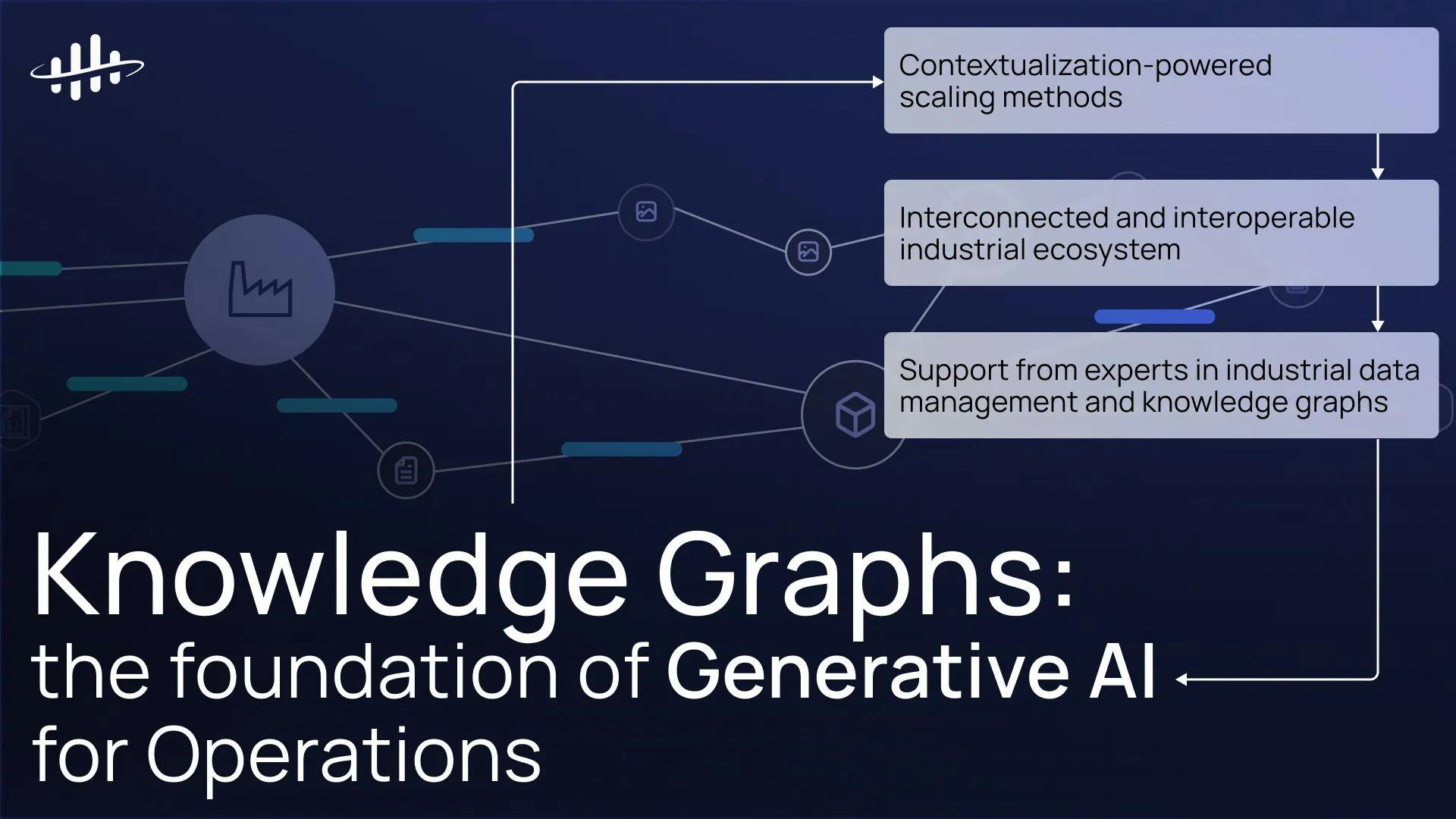
As generative AI begins to redefine the industrial landscape, digital transformation leaders continue to grapple with the challenges of deciphering complex, unstructured data, defining precise use cases, and showcasing immediate business value as they race to implement AI solutions.
Amidst these challenges, the industrial knowledge graph emerges as a strategic solution. Knowledge graphs, as we will detail below, provide the essential foundation generative AI requires by improving data utilization and breaking down data silos. As such, the industrial knowledge graph becomes the linchpin for unlocking tangible business value in the age of generative AI.
Understanding Knowledge Graphs
At its core, a knowledge graph is a structured representation of information that allows industrial organizations to better understand the relationships and connections within complex datasets. These data relationships are made possible through contextualization pipelines that help create and maintain a dynamic industrial knowledge graph. Thus, by leveraging access to contextualized data, knowledge graphs address three key challenges:
- Overcoming Data Silos: In industrial settings, data often resides in numerous silos, leading to duplication and ambiguity in meaning. Knowledge graphs play a pivotal role in breaking down these silos, providing a unified view of data, and improving the understanding of usage and consumption patterns.
- Unleashing Unstructured Data: By employing standardized metadata, the knowledge graphs allow for the categorization and management of information, enhancing the utilization of unstructured data present in documents, images, and videos (another common data silo) and turning that data into actionable insights.
- Enhancing Business Insights: The explicit contextualized knowledge, rules, and semantics embedded in knowledge graphs empower AI applications to provide high-quality, trusted insights that are absolutely necessary for working industrial domain and allow subject matter experts to make high-quality decisions, enhancing business processes, workflows, and operations.
Importance of Knowledge Graphs in Enabling Generative AI for Operations
According to Gartner's Emerging Tech Impact Radar, Generative AI report, knowledge graph adoption has rapidly accelerated with the growing use of AI because knowledge graphs provide the explicit knowledge, rules, and semantics needed in conjunction with AI/ML methods for pattern recognition. In other words, knowledge graphs deliver trusted and verified data to Large Language Models (LLMs) and provide rules to contain the model. Having access to trusted and verified data is particularly important when LLMs are used with Retrieval Augmented Generation (RAG). This design pattern helps provide contextualized industrial data directly to the LLM as specific content to form a trustworthy, deterministic response.
In this way, knowledge graphs are a key underlying technology and act as the backbone for generative AI solutions across business functions that will drive business impact, including:
- Digital workplace (e.g., collaboration, sharing and search)
- Automation (e.g., ingestion of data from content to robotic process automation)
- Data Exploration (e.g., providing deeper insights into structured and unstructured data)
- Data management (e.g., metadata management, data cataloging, and data fabric)
Despite the undeniable benefits of knowledge graphs, Gartner identifies several challenges to successful implementation. Let’s take a look and see how we can address these challenges:
Challenge 1: Immature Scaling Methods: As knowledge graphs transition from prototypes to production, methods to maintain their scalability while ensuring reliable performance, handling duplication, and preserving data quality are still evolving.
Solution: To provide reliable performance and scalability, organizations must ensure (as mentioned earlier) that their knowledge graphs are powered by contextualization services in order to provide high-quality, trusted insights that lead to higher levels of adoption by the teams across the enterprise.
Challenge 2: Interoperability: Enabling internal data to interact with external knowledge graphs (meaning connecting data and graphs that vary in scope, ownership, data types, etc,) seamlessly remains challenging. Overcoming this hurdle is vital for creating a truly interconnected and interoperable industrial ecosystem.
Solution: To establish and maintain interconnection and interoperability, we need to ensure that there is access to fully documented and open APIs that help facilitate connections between different data systems, industry standards models, or third-party applications. Plus, having strong contextualization capabilities ensures the necessary background for meaningful integration and interpretation of information, especially when that information is trapped in a siloed data source.
Challenge 3: Scarcity of In-House Expertise: Particularly among small and midsize businesses, expertise in knowledge graphs is scarce. Identifying and accessing third-party providers with the necessary proficiency becomes a significant obstacle.
Solution: Working with a third-party provider with expertise in building industrial knowledge graphs and industrial data management should not be that scary, especially if you know what to avoid during decision-making when purchasing software and what software deployment type works best for your organization and goals.
A knowledge graph enables an industrial organization to extract value from unstructured and siloed data sources. Establishing a dynamic and interoperable industrial knowledge graph with access to high-quality contextualized data must be the first step for any organization that wants to implement generative AI initiatives that improve operations and accelerate time to value.
Learn more
To learn more about making generative AI work for industry, read The Definitive Guide to Generative AI for Industry, a free resource authored by AI innovators at Cognite.
See Cognite Data Fusion® in action
Get in touch with our product experts to learn more and identify quick wins